Our paper entitled “A Distributed Multi-Sensor Machine Learning Approach to Earthquake Early Warning” was selected in the Artificial Intelligence for Social Impact track at AAAI-20. This work addresses the challenges of characterizing earthquake and issuing broadcast alerts in a matter of seconds by relying on an effective use of scientific instruments and large-scale detection.
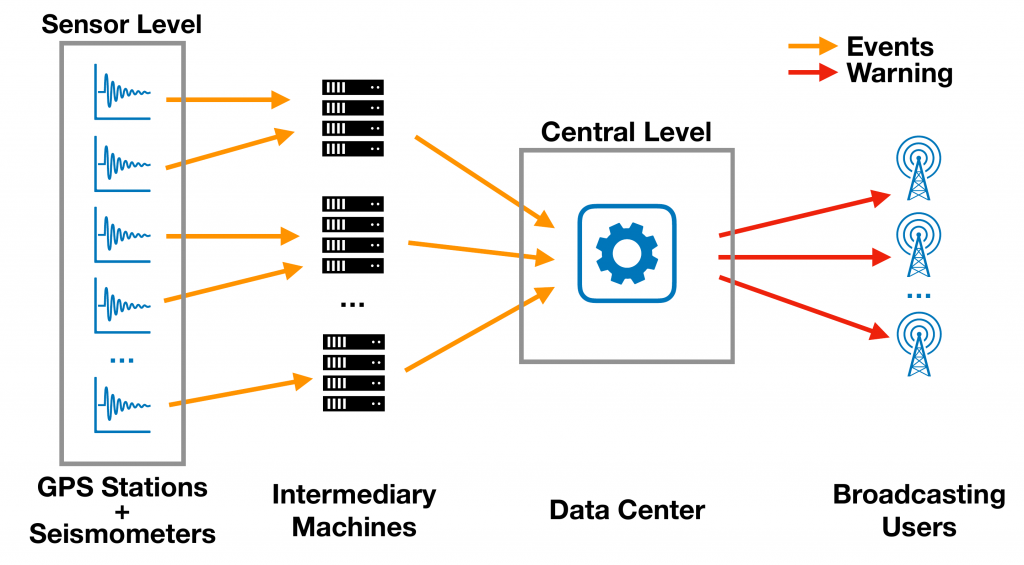
As categorizing medium earthquakes is not done well by GPS, and categorizing large earthquakes is not done well by seismometers, the paper proposes a predictive model that combines these measurements using machine learning techniques to improve the accuracy of Earthquake Early Warning systems. Specifically, the authors proposed a Distributed Multi-Sensor system that combines data from both types of sensors (GPS stations and seismometers) to detect medium and large earthquakes at scale.
Evaluations on real-world earthquake datasets have shown to be more accurate than their baseline while being robust to infrastructure failures.
The project is based on an interdisciplinary research collaboration between RDI2, the INRIA Large-Scale Collaborative Data Mining (LACODAM) team in France, and Univ. of Oregon.
Preprint: https://hal.archives-ouvertes.fr/hal-02373429/document